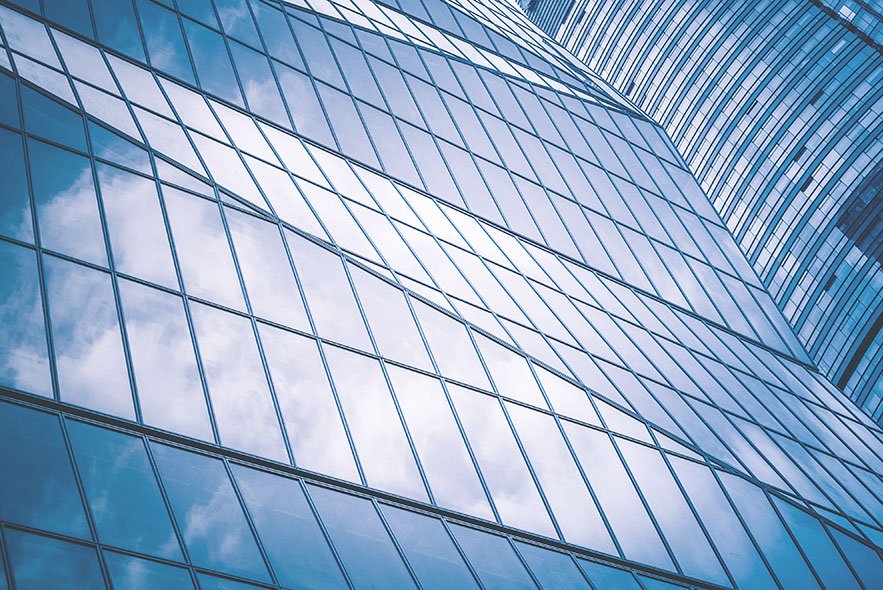
conversational AI
What is conversational AI?
Conversational AI is a type of artificial intelligence that enables computers to understand, process and generate human language.
Conversational AI has primarily taken the form of advanced chatbots, or AI chatbots. In distinction to conventional chatbots, which are predicated on simple software programmed for limited capabilities, AI chatbots combine different forms of AI for more advanced capabilities. The technologies used in AI chatbots can also be used to enhance conventional voice assistants and virtual agents. The technologies behind conversational AI are nascent, yet rapidly improving and expanding.
A conversational AI chatbot can answer frequently asked questions, troubleshoot issues and even make small talk -- contrary to the more limited capabilities that exist when a person converses with a static chatbot with narrow functionality. A static chatbot is typically featured on a company website and limited to textual interactions. In contrast, conversational AI interactions are meant to be accessed and conducted via various mediums, including audio, video and text.
Examples of conversational AI
Prominent types of conversational AI include the following:
- All-encompassing, subscription-based chatbots. These advanced chatbots can generate text to answer user queries on a broad range of topics. Foremost among them is OpenAI's ChatGPT. OpenAI requires users to present login information to interact with the application, and paid subscriptions are available.
- AI-powered search engine assistants. A search tool with AI functionality can quickly produce a search result or results that best match a user's query. The most prominent examples include, but aren't limited to, Google's Bard and Bing AI.
- Conversational business intelligence (BI) apps. Conversational BI combines conversational AI with data analytics capabilities, allowing users to, in a sense, talk to these applications and receive outputs in the form of data visualizations and explanations. A conversational BI application is integrated with a database or data warehouse from which it retrieves data needed for analysis and visualization.
- Customer service chatbots. The most well-known chatbots and virtual assistants are on company websites, offering limited responses to customer inquiries and some predetermined functionality. Virtual assistant platform vendors offer services to help businesses better engage their customers. IBM Watson Assistant is one prominent example.
How does conversational AI work? Processes and components
Conversational AI works by combining natural language processing (NLP) and machine learning (ML) processes with conventional, static forms of interactive technology, such as chatbots. This combination is used to respond to users through interactions that mimic those with typical human agents. Static chatbots are rules-based and their conversation flows are based on sets of predefined answers meant to guide users through specific information. A conversational AI model, on the other hand, uses NLP to analyze and interpret the user's human speech for meaning and ML to learn new information for future interactions.
NLP processes large amounts of unstructured human language data and creates a structured data format, through computational linguistics and ML, so machines can understand the information to make decisions and produce responses. An ML algorithm must fully grasp a sentence and the function of each word in it. Methods like part-of-speech tagging are used to ensure the input text is understood and processed correctly.
Two subtopics of NLP play a crucial role in conversational AI: natural language understanding (NLU) and natural language generation (NLG).
- NLU is what enables a machine or application to process the language data in terms of context, intent, syntax and semantics, and ultimately determine the user's intended meaning.
- NLG is the process by which the machine generates text in human-readable languages, also called natural languages, based on all the input it was given. The goal of an NLG system is to explain the AI's structured data for humans to understand.
Real-world benefits and challenges of conversational AI
Conversational AI is expanding and offering benefits to many industries, including the following:
- Healthcare. Conversational AI can help patients describe their conditions online through a series of questions meant to circumvent wait times.
- Retail. When customer service representatives aren't available, AI-powered chatbots are able to meet customers' demands on a 24/7 basis, even during holidays. Historically, call centers and in-person visits were the only way to interact with customers. Now, customer support is no longer limited to office hours, because AI chatbots are available through various mediums and channels, including email and websites.
- Banking. Bank personnel can have AI chatbots handle complex requests in a manner that conventional chatbots would struggle with. When dealing with customers' finances, it's especially important to eliminate common human errors and deliver precise and accurate responses or solutions to address their concerns.
- IoT. Common household devices, such as Amazon Echo and Apple's Siri, have conversational AI capabilities. Conversational AI agents can even be interacted with through smart home devices.
- Human resources. Conversational AI can automate the time-consuming HR process of sifting through candidate credentials manually during recruitment for a job.
There are some challenges with conversational AI development. Conversational AI models have thus far been trained primarily in English and have yet to fully accommodate global users by interacting with them in their native languages. Companies that conduct customer interactions via AI chatbots must have security measures in place to process and store the data transmitted. Finally, conversational AI can be thrown off by slang, jargon and regional dialects, which are all examples of the changing nature of human languages. Developers must train the technology to properly address such challenges in the future.