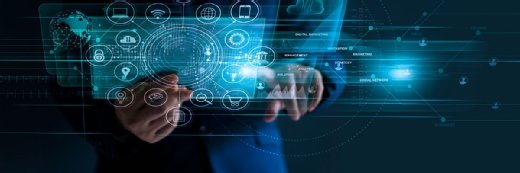
Getty Images/iStockphoto
Dremio adds first generative AI-infused tool, intros others
The vendor's initial generative AI-infused tool is Text-to-SQL, which enables customers to work with data using natural language that automatically gets translated to code.
Dremio launched its first generative AI-infused tool and introduced two others, all aimed at making users more efficient and speeding the process of deriving insights from data.
The vendor unveiled Text-to-SQL on June 15; it is now generally available. Autonomous Semantic Layer and Vector Lakehouse were also revealed the same day but are still in preview.
Based in Santa Clara, Calif., Dremio is a data lakehouse vendor whose platform combines the structured data management capabilities of data warehouses with the unstructured data management capabilities of data lakes. Databricks, among others, offers a similar data lakehouse platform.
Before unveiling its first tool integrating generative AI and large language model technology -- and plans for two additional tools featuring generative AI -- in March 2022 Dremio launched a new query engine and introduced its new metadata management service.
Three months earlier, the vendor raised $160 million in venture capital funding to bring its total funding to $410 million.
The first of three
Text-to-SQL is designed to improve users' productivity, according to Tomer Shiran, founder of Dremio and the vendor's chief product officer.
Using the LLM capabilities of generative AI, the tool enables users to type queries in natural language rather than code and then converts the natural language into code that Dremio's technology can understand.
The concept of translating natural language to code is not new. For example, analytics vendor ThoughtSpot built its entire platform around natural language search.
But until the release of ChatGPT in November 2022 -- which represented a substantial boost in the power of generative AI and LLMs -- the vocabularies of natural language tools were unsophisticated and despite their best intentions still required data literacy on the part of the user.
Now, however, generative AI and LLM tools have much more extensive vocabularies and enable freeform natural language interaction.
Data observability vendor Monte Carlo recently launched tools that involve converting natural language to SQL -- and the reverse -- to make data engineers using the vendor's platform more efficient. Now Dremio's Text-to-SQL is poised to do the same for its users, according to Stewart Bond, an analyst at IDC.
"AI based on large language models is opening new opportunities for changing the user experience and increasing productivity," he said. "Dremio's Text-to-SQL is an example that we are seeing in the database market, and we have seen elsewhere."
Tools such as Text-to-SQL are part of the first wave of data management and analytics features to incorporate generative AI, doing the straightforward task of translating between natural language and code, Bond added.
As generative AI continues to evolve and product development teams have more time to build features, more sophisticated applications will emerge.
Shiran similarly said that the promise of Text-to-SQL is more efficiency.
Not only does translating between natural language and code potentially enable people without extensive data literacy training to work with data but it also could save data experts time as they do their work.
Without having to write code for every query -- and find and fix code when there's the even a small mistake -- data experts will be freed from certain monotonous tasks and able to do more in-depth analysis.
"With every person able to see their data within seconds, users can focus on their data rather than getting their data," Shiran said.
Two to come
While Text-to-SQL is now available, Autonomous Semantic Layer and Vector Lakehouse are still in the preview stage.
Autonomous Semantic Layer aims to automate the cumbersome process of modeling and cataloging data. To make data accessible and usable, all data is defined and categorized as it is ingested and integrated.
Those semantics -- the definitions and categorization -- make data points and datasets easier to find amid countless other data points and thousands of datasets. In addition, they make relationships between data points and datasets easier to discover.
But defining and categorizing data is monotonous and time-consuming.
Dremio, therefore, is developing Autonomous Semantic Layer with generative AI to automate much of data cataloging that otherwise has to be done manually. The generative AI technology automatically learn the details of users' data and develops the semantic descriptions of datasets, columns and relationships.
The result is that data engineers no longer have to manually catalog all of their organization's data. The tool does it for them.
As with Text-to-SQL, the aim of Autonomous Semantic Layer is to improve efficiency to ultimately reach insights more quickly, according to Shiran.
"Data teams spend most of their time getting their data cleaned, documented and prepared, which slows down the time to insight," he said. "The Autonomous Semantic Layer aims to solve this problem through automatic documentation, automatic data acceleration and semantic search."
Vector Lakehouse, meanwhile, is designed to improve semantic searches, recommendation systems and anomaly detection, according to Shiran.
He noted that one of generative AI's unique capabilities is its ability to automatically create vector embeddings -- numerical representations of unstructured data such as text, images and videos. Those representations essentially brings structure to the unstructured data points, which enables them to be combined with structured data, categorized for discoverability and ultimately used for analysis.
Without generative AI, data teams are generally forced to deploy standalone vector databases, leading to isolated data repositories. But by combining Dremio's data lakehouse platform with generative AI, the vendor aims to enable customers to store their unstructured data along with their structured data for easier access and more efficient analysis.
"Companies will be able to manage and process [vector] embeddings alongside source data in an open data lakehouse architecture," Shiran said.
Future plans
The combination of generative AI with data management and analytics capabilities is in its nascent stage, as Bond noted.
Therefore, there's no specific set of generative AI-infused capabilities that Dremio and all other data management vendors need to build in order to remain competitive. Each is in its exploration phase and coming up with its own ideas.
"We are still very early days," Bond said. "But there is a lot of opportunity for what will be possible."
Tagging and classification -- which Dremio says it's addressing -- is one area that can benefit significantly from generative AI, he continued. So is using natural language to not only translate queries into code but also to develop entire data pipelines.
Meanwhile, "Dremio's roadmap is centered around cost efficiency and speed-to-insight," Shiran said of the vendor's plans.
One tool the vendor has under development that aims to help organizations control their cloud computing costs is Dremio Artic. It is a lakehouse catalog designed to reduce infrastructure costs by eliminating the need to replicate data across development, testing and production environments.
When the same data is needed in three different environments, costs triple.
So Artic, which provides users with branches within the same environment rather than forcing them to build three separate cloud environments, has the potential to significantly lower organizations' cloud computing costs, according to Shiran.
Eric Avidon is a senior news writer for TechTarget Editorial and a journalist with more than 25 years of experience. He covers analytics and data management.